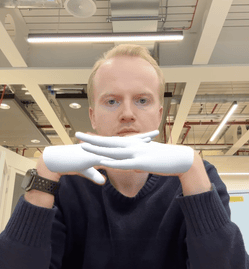
3D Hand Tracking
Lens Studio, Mobile Devices, Web
Snap Inc.
Computer Vision Engineer at Snap
Building machine learning models that facilitate billions of user interactions at Snap.
Snap Inc.
PhD Thesis
I obtained a PhD degree under the supervision of Prof. Michael Bronstein and Prof. Stefanos Zafeiriou in the Department of Computing at Imperial College London.
Dominik Kulon, Riza Alp Güler, Iasonas Kokkinos, Michael Bronstein, Stefanos Zafeiriou
We introduce a simple and effective network architecture for monocular 3D hand pose estimation consisting of an image encoder followed by a mesh convolutional decoder that is trained through a direct 3D hand mesh reconstruction loss. We train our network by gathering a large-scale dataset of hand action in YouTube videos and use it as a source of weak supervision. Our system largely outperforms state-of-the-art methods, even halving the errors on the in-the-wild benchmark.
Dominik Kulon, Haoyang Wang, Riza Alp Güler, Michael Bronstein, Stefanos Zafeiriou
Monocular 3D reconstruction of deformable objects, such as human body parts, has typically been approached by predicting parameters of heavyweight linear models. In this paper, we demonstrate an alternative solution that is based on the idea of encoding images into a latent non-linear representation of meshes.
MRes Thesis
The thesis presents a system that establishes a correspondence from hand pixels in an RGB image to the 3D hand model in an end-to-end manner. It is accompanied by a hand model capable of representing different shapes learned from hand scans and the first dataset of 1,500,000 hand models optimized to match the pose and shape of hands in color images.